报告主题简介:
In the era of big data, conventional computers are increasingly challenged by the approaching end of Moore’s law, and by the latency/energy burdens of moving data between memory and processor in the von Neumann architecture. To get around these fundamental limits, in-memory computing (IMC) has recently emerged as a promising technique to conduct computing in situ, i.e., within the memory unit. One typical IMC methodology is based on resistive memories, such as resistive switching memory, phase change memory and magnetic memory. By exploiting the crosspoint memory architecture or the resistive switching dynamics, various IMC schemes can be realized.
Here we first show that a crosspoint array of analog resistive memories can directly solve a system of linear equations, or find the matrix eigenvectors. These computations are completed in just one single step, thanks to the physical computing with Ohm’s and Kirchhoff’s laws, and thanks to the feedback connections in the crosspoint circuit. Representative algebraic problems are demonstrated in hardware and applied to classical computing tasks, such as implementing Google’s PageRank and solving the Schrödinger equation in one step. Then, we introduce the concept of stateful neural network, which performs all Boolean logic operations with the same circuit topology of resistive switches. Stateful neural network can solve all 2-input logic operations with just one step, except for the XOR needing two sequential steps. 1-bit full adder is realized with just two steps and five resistive switches, thus highlighting the high efficiencies of space, time, and energy of the stateful neural network for logic computing.
报告人简介:
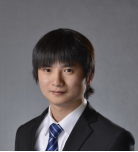
孙仲,2011毕业于南开大学材料物理系,获理学学士学位;随后进入清华大学物理系学习,于2016年获理学博士学位。目前在意大利米兰理工大学从事博士后研究工作。主要从事新型存储器及存内计算、机器学习芯片等方面的研究,在新型存储器物理机制,存内逻辑计算、模拟计算和机器学习等领域开展了多项开拓性研究,取得了多项创新成果。近三年的研究成果发表在PNAS、Nat. Commun.和Adv. Mater.等国际顶级期刊上,并得到国际媒体的广泛关注和报道。